About Me
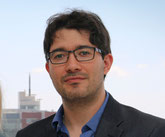
My research involves the use of machine learning/statistical methods (e.g. neural networks, kernel methods) to downscale (statistically refine) the coarse-resolution reanalysis and global climate model outputs (GCM) to finer, local scales. I am also interested in supervised and unsupervised classification methods, like self-organized maps, and their application to extract features of big datasets. In general, I am passionate about nonlinear time series analyses and enjoy using novel methods to improve our predictive skill of different phenomena.
Applications include climate change projections of maximum and minimum temperatures, precipitation occurrences and amounts, wind speeds, and extreme weather projections for different climate change scenarios. Most recently my co-authors and I used Bayesian Neural Networks to predict maize yield in China.